Iteratively Reweighted FGMRES and FLSQR for sparse reconstruction
With Silvia Gazzola
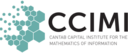
Iteratively Reweighted FGMRES and FLSQR for sparse reconstruction
Krylov subspace methods are powerful iterative solvers for large-scale linear inverse problems, such as those arising in image deblurring and computed tomography. In this talk I will present two new algorithms to efficiently solve L2-Lp regularized problems that enforce sparsity in the solution. The proposed approach is based on building a sequence of quadratic problems approximating the original L2-Lp objective function, and partially solving them using flexible Krylov-Tikhonov methods. These algorithms are built upon a solid theoretical justification for converge, and have the advantage of building a single (flexible) approximation (Krylov) Subspace that encodes regularization through variable “preconditioning’’. The performance of the algorithms will be shown through a variety of numerical examples. This is a joint work with Julianne Chung (Virginia Tech), James Nagy (Emory University) and Malena Sabate Landman (University of Bath).
- Speaker: Silvia Gazzola
- Wednesday 15 July 2020, 14:00–15:00
- Venue: Virtual Zoom meeting.
- Series: CCIMI Seminars; organiser: J.W.Stevens.